8 Trends in High-Performance Analytics – Insights from Forrester
Forrester, as a guest of Kinetica, held a webinar this month about how digital business is driving rapid expansion and sophistication of data and insight, and why it’s necessary to augment traditional CPU-bound analytics with GPUs, in-memory processing, and machine learning. Michele Goetz, Principal Analyst at Forrester Research, and Kinetica’s Manan Goel, VP of Products at Kinetica, talked about this transformation in detail and how a modern GPU-accelerated, in-memory analytics database converges Machine Learning and OLAP, and delivers real-time insights to get to decisions and actions faster.
Here are some key industry observations made by Principal Forrester Analyst Michele Goetz:
Digital Transformation Background
Let’s put the term “digital transformation” into a bit of context in terms of how it can help drive your insight-driven business:
Digital isn’t just a buzzword. Digital is about the ability to use digital insights to transform your business. It really is a transformative capability within your business. The core tenant of digital transformation is the fact that you can go from data, to insight, and to action very quickly. It really speeds up your business to be much more competitive within your industry and your market.
Going digital helps you harness that insight, wherever it comes from. You need to be there in the moment so that any sort of data and insight that is flowing in doesn’t perish. You capitalize it; you get some value out of it when you need it and when that value is realized. Lastly, in the cycle of interacting with insight in a digital environment, everything becomes highly personalized. Everything is relevant to what you’re trying to achieve at a particular moment, as well as what your strategies will be in the future. Ultimately, what digital is about is turning your insight into action to achieve real business results.
Going digital is both a strategy as well as an aspiration. There are many barriers that exist to get us to the point where we’re truly successful and getting real business value. In terms of digital data, you need to think about processing the vast amounts of data and data varieties, as well as supporting the performance levels needed for extreme services and queries. With CPU architectures, those performance levels aren’t always satisfied and are bound to the processing capacity of the CPU.
A lot of bottlenecks can occur in this process. Bottlenecks can occur not only in the way that we process data, but in how we’re providing the support for the extreme number of services and queries that must run on these systems.
We need to be able to render analytic visualizations in real time. We’re starting to tell stories with our data in ways that are very different than the typical kind of interactions we’ve had via application systems. A lot of the information that we are receiving is coming in a visual format, and we need to be able to render our visualizations in real time. Traditional systems were not intended to support this; they were intended to process transactions.
We are working in an extremely hybridized world, spanning on-premise, cloud, and external data. We need to be able to harness a lot of the scale, capabilities, and lower costs of the cloud. We also need to bring in external information that we can harness, whether that’s weather data, geo data, or customer data. This will help us to augment our understanding about not only our customers, but what’s happening within the market. The key message is that it can be complicated to ingest data across such a variety of ecosystems and platforms.
Organizations need to operate in a such a way that they can meet their service level requirements while operating within their budget. In many cases, the siloed, monolithic systems that companies have been building from an enterprise perspective have been very bespoke, purpose-built, and chained to only one type of delivery mode, data processing mode, and management mode. We need to be able to have a high-performance environment that we can use when we need it, and move into a slower, lower-cost environment when appropriate.
8 Trends in High Performance Analytics
1. 71% of organizations are planning to implement, have implemented, or expanded advanced visualization capabilities. What does this mean for high computing environments and where companies are starting to invest? A lot of investment is happening around advanced visualization. How do you tell better stories? How can you see information in a way that you recognize the insight immediately, rather than trying to analyze large volumes of data and facts from a report or a spreadsheet?
As an example, weather data is becoming increasingly incorporated into our insights and visualizations. It’s done in a way that we wouldn’t necessarily think of before. For example, weather data and visualizations are helping to:
- Optimize retail store resources
- Predict shopping behavior
- Increase oil production
- Understand property value
- Optimize traffic flow
Weather information can help retail stores optimize the types of resources that they’re going to have on hand. Principal Forrester Analyst Michele Goetz brought up Walmart as an example. If it’s going to be a beautiful weekend, then Walmart knows that a lot of people are going to barbecue and start to do more gardening. As a result, Walmart knows that the traffic in the store is going to require more hands on deck and more checkout lines. On the other hand, when you have a snow storm, you may just have a skeleton crew.
2. 51% of organizations are investing in distributed, real-time insight delivery technology. More and more companies are thinking about how to transition the analytics and insight that they’re doing and pour that into events, actions, and business processes, so that they can capitalize on opportunities immediately. Not only are the level of analytics increasing in sophistication, but you can also bring in predictive location or text analytics. The other important point is that you can perform stream analytics; you can analyze information coming in as you’re trying to understand the environment around you. You’re taking the analysis that you’ve done to recognize historical patterns. You want to stream your algorithmic model together so that you know what types of actions should be taken.
3. 51% will combine content management and data management programs into a unified information management program. We’re really seeing a lot of consolidation between data management and information management. This uniformed environment helps in areas such as insurance and claims processing. How are the adjusters’ notes coming in as they take a look at accidents that may have happened or damage that has been done to property? What are the notes that are coming in from law enforcement around what happened during the accident? The merging of those types of information require much more sophisticated analytics. You want to be able to visualize this information so that you can understand what to do next; having a unified capability to process all this information is really quite critical.
4. 36% of mobile executives use location and other contextual information to deliver the most relevant service or content. It’s important to be able to understand how digital is really reshaping the way that we engage internally within our organization, as well as externally with our partners and customers. You can’t underestimate how much mobile is starting to impact the way we do business and the way that we run our lives. For mobile executives, we’re thinking about mobile as a way to deliver applications and experience. But you realize very quickly that there are a number of micro-services that are underpinning the apps that are being developed. We can also embed visualization within these apps to provide information, and this means that we need to be able to combine data and analytics and produce this insight in real time. This information has to be quickly delivered across highly sophisticated and orchestrated micro-services to create those experiences, and to provide value in these mobile environments. Not only are mobile executives thinking about context and location information for content delivery, but they’re also recognizing the fact that data really does provide that interactive experience.
5. HPC GPU platforms are needed to meet the challenge. GPU platforms are able to:
- Process structured, semi-structured content and media
- Take advantage of cloud and hybrid cloud ecosystems
- Power advanced analytic workloads
- Run artificial intelligence and machine learning alongside business intelligence
- Allow for analyst self-service playgrounds for rapid training of models and visual exploration
6. GPU platforms allow you to move heavy-duty processing processing to wherever you want, based on computing performance requirements. This is where your platform is really going to support your digital business. First, it’s going to be able to process all types of information; you’re not just constrained to structured, transactional information. You can merge streaming video and audio data with social information, as well as any transactional or institutional data that you may have. You can move different computing performance requirements across cloud or hybrid cloud ecosystems, and high performance computing takes that into account. With GPU platforms, you can move the heavy-duty processing to the place that makes the most sense based on what your performance and service level agreements are going to be.
7. GPU platforms can also power more advanced analytic workloads. Think about the amount of information and the number of simulations that you can run in real time. GPUs really power that type of environment. GPUs can also give you a leg up if you’re starting to consider things like artificial intelligence and machine learning. You can use GPUs to take your AI and machine learning capability and sit that alongside the business intelligence from your daily transactions, business processes, and engagement with your customer.
8. GPU platforms are helping to assist in “playgrounding” efforts. In the past, you just needed an environment that you could hammer on and take in a lot of the querying and servicing of the data as you’re running it through analytics. But much more of the analytics is evolving into more sophisticated algorithms. There is a lot more training that is involved and a need for more visual exploration of the data to train and vet the more sophisticated algorithm models that you’re doing, particularly around AI and machine learning. This is really what GPUs are intended to be able to support.
Summary
Fast analysis is critical for decision makers. To meet this need, organizations are becoming insight-driven and are transitioning from traditional systems to GPU platforms in order to harness vast amounts of data, benefit from richer visualizations, and perform analytics in real time. In today’s hybridized world of on-premise, cloud, and external data, it’s more important than ever to be able to ingest data across such a variety of ecosystems and platforms. GPUs can help you quickly visualize your data and take action on those insights. With HPC and GPUs, you can move away from the simple graphs and charts that you’ve normally used to tell stories, and benefit from rich visualizations so that you can make decisions and actions faster.
Making Sense of Sensor Data
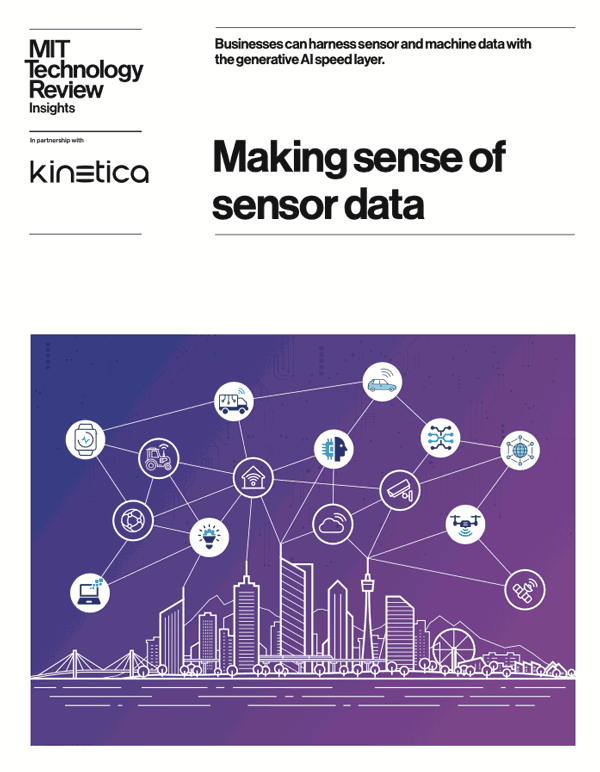