6 Ways GPU Acceleration is Disrupting Financial Analytics
Next-generation GPU computing is opening new opportunities for intelligent, real-time analytics within the Financial Services industry.
It is widely reported that in the early 1800s, the Rothschild banking family of France set up a vast network of pigeon lofts spread across Europe, and deployed a prized coop of racing pigeons to fly between its financial houses, carrying the latest news. From the pigeons, Nathan Rothschild was reportedly the first to learn of the British victory at Waterloo. The story goes that while other traders on the stock exchange prepared for a British loss, he went long and made millions. At the time, pigeons were the fastest way to send and receive information ahead of the competition.
Fast forward a few hundred years, and the financial services community is still on the forefront of using newer and more sophisticated technologies for competitive advantage. Banks, credit card companies, hedge funds, brokerages, and insurance firms are all aggressively testing and deploying the latest cutting-edge technologies to enable real-time analytics and AI use cases. Their primary goals are to reduce risk, execute smarter trades, increase profitability, and eliminate fraud.
Keeping up with the volume of data and the complexity of analysis has not been easy. Organizations cannot be competitive while waiting for slow queries or overnight batch reports. Legacy databases and analytics tools have maxed out their capabilities. A next-generation solution is required.
Enter parallel processing with GPUs (Graphics Processing Units). Traditionally, analytics applications have used the CPU for computation. CPUs process data sequentially, and have only a handful of cores to work on tasks. A new breed of high-performance analytics applications are now being built to process data with GPUs. The typical GPU has over 4,000 processing cores and computation-heavy tasks can be designed to run in parallel on these processors.
These new applications must be specifically designed to take advantage of the parallel compute capabilities of the GPU. Kinetica is such an example – a GPU-accelerated analytics database, built from the ground up, for analytics and machine learning on large and streaming datasets. It leverages the GPU to deliver performance improvements that were previously impossible.
- Advanced Risk Calculations in Milliseconds Delivering real-time and intra-day risk management analytics have been major technical challenges for financial organizations. Kinetica is able to perform risk calculations and simulations in near real-time using up-to-the-moment data. An in-database analytics API allows for custom functions, such as Monte Carlo simulations, to run in-database on the GPU. These advanced capabilities are leading to better informed investment decisions, and enable organizations to react with more confidence to market events, while reducing risk.
- Real-Time Sentiment Analysis Quantitative traders are turning to sentiment indicators from social media, supply chain analysis, news, company forums, and even satellite data to inform trading models. NLP and machine learning methods can be put to work on these text-heavy and geo-temporal datasets to identify opportunities and optimize alpha.
- Fast Query Supports Efficient Compliance The ad-hoc query demands of regulatory compliance have often been slow and cumbersome on the large datasets involved. Customers are seeing opportunities to proactively stay ahead of compliance concerns, while enabling fast reporting on a massive scale.
- Parallel Compute Power for Identifying Fraud and Abuse The diverse and high-cardinality datasets typically monitored for fraud pose particular difficulties, as they are hard to index. GPUs provide enough brute force that indexing is less important, resulting in vast improvements when looking for anomalies in diverse streams of transaction and log data.
- Real-Time Personalization & Customer 360 Consumer banks are building targeted personalization offers through analyzing every aspect of customer behavior. This data comes from many channels: transaction data (online, branches, ATMs), social media posts, customer service records and location data. The goal is to build brand loyalty with tailored content and services for each customer. It is being used to send real-time, targeted marketing offers, cross sell/up-sell opportunities and increase customer brand loyalty. A GPU database provides the processing power needed for the high speed ingest, processing and real-time analysis.
- Faster Credit Scoring and Risk Modeling Traditional credit scoring analytics and modeling applications built on legacy CPU technologies are not keeping pace with today’s business requirements. Accelerating these models and leveraging deep learning neural networks on the GPU result in more accurate predictive analytics, and in most cases, 100x faster risk modeling performance.
The financial services industry is one of the most data-driven industries in the world. But data itself doesn’t inherently have value; how the data is used and how fast the data can be analyzed are the two core components of creating a competitive advantage in finance. GPUs, combined with advanced analytics, can help your business drive revenue and push your organization across the finish line long before the rest of the “pigeons” come home to roost.
For a more in-depth look at GPU databases and how they are creating new opportunities, take a look at our eBook: How GPUs are Defining the Future of Data Analytics or Contact us for a demo today!.
Also:
Making Sense of Sensor Data
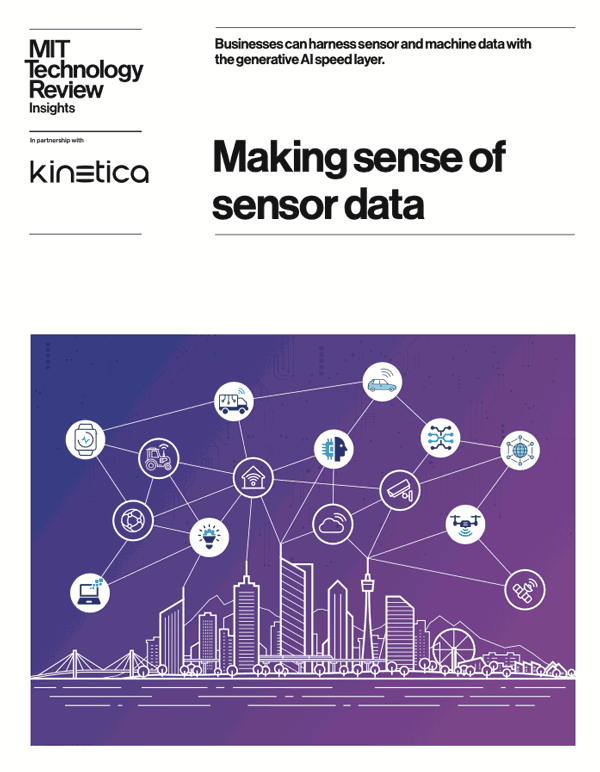