While artificial intelligence and machine learning give corporations the ability to act faster with fewer employees than ever before, companies take on a new kind of unprecedented risk, as well. AI and ML algorithms are only as good as the data they learn from. If they are trained to make decisions using a data set that doesn’t include enough examples or biased examples of certain groups of people, they will produce unintentionally biased results—or worse, decisions, as appears to be the case with the Apple Card.
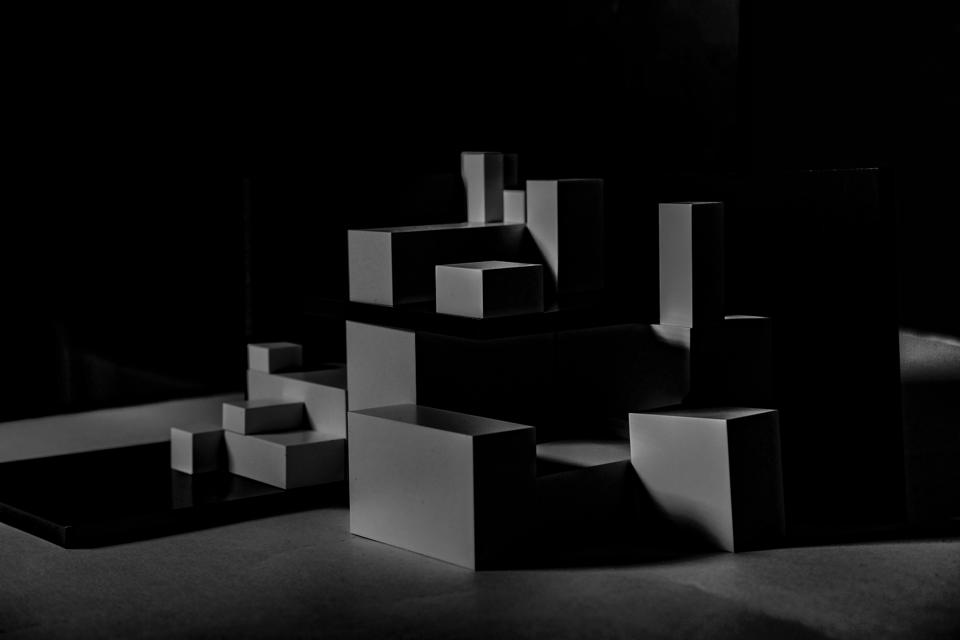
If a collection of images showed many examples of women in the kitchen, for example, an algorithm trained using that collection of image data would form an association between women and kitchens that it could reproduce in its assumptions and decisions.
The parameters of what the algorithm should take into account when analyzing a data set are still set by people. And the developers and data scientists doing this work may not be aware of the unconscious biases the parameters they’ve put in place contain. We don’t know what the parameters were for the Apple Card’s credit determinations, but if factors included annual income without considering joint property ownership and tax filings, women, who in America still make 80.70 for every man’s dollar, would be at an inherent disadvantage.
To date, our efforts to establish equal protection under the law have been aimed at preventing conscious human bias. The problem with AI is that it reproduces our unconscious biases faster and more effectively than we ever could, and it does so without a moral conscience—or concern for PR. The algorithm, in this case, meted out credit decisions without the higher order thinking skills that would lead a human to see a red flag in the stark differences between the credit limits offered to women versus men on the whole.
Part of the problem here is that, as with many AI and ML algorithms, the Apple Card’s is a black box; meaning, there is no framework in place to trace the algorithm’s training and decision-making. For corporations, this is a significant legal and PR risk. For society, this is even more serious. If we cede our decision-making to AI, whether for ride-sharing refunds, insurance billing, or mortgage rates, we risk subjecting ourselves to judgment with no appeal, to a monarchy of machines where all the world’s a data set, and all the men and women, merely data.
We don’t have to accept this new world order. We can innovate in a responsible way. We need to ensure that the new crop of machine learning-enabled data platforms have the necessary infrastructure to implement governance, transparency, and repeatability. Along with that infrastructure, we need a framework to trace the lineage of an algorithm’s training. Singapore’s Personal Data Protection Commission has begun to tackle this issue by creating the Model AI Governance Framework, and The World Economic Forum is working on an initiative to make the framework accessible to corporations and governments across the globe.
We can always determine whether or not outcomes reflect our social values and meet our legal standards. But if we can assess the data that goes into training a model beforehand, and continuously evaluate that model’s performance, then we can find the flaws in the system that produce unintentional bias and fix them—before we have to hear about them on Twitter.
Irina Farooq is chief product officer at Kinetica. You can follow her on Twitter @IrinaFarooq.